UniDL4BioPep: A universal deep learning architecture for bioactive peptide prediction
The webserver is the implementation of the paper "Du, Z., Ding, X., Xu, Y., & Li, Y. (2023). UniDL4BioPep: a universal deep learning architecture for binary classification in peptide bioactivity. Briefings in Bioinformatics, bbad135."
Notice: For very large dataset processing: please download our model locally or contact us at zhenjiao@ksu.edu or yonghui@ksu.edu for more assistant.
Usage of the webserver:
Example for “Quick output version” :
1. Select “Antihypertensive” model for antihypertensive activity prediction. → → → 2. Insert a peptide or protein sequence, “VPP” → → → 3. Click “Run”→ → → 4. The result will be returned in seconds below the “Run” button
Notice: it also support multiple sequence at the same time. Just input as “VPP,IPP,CCL,AGR” (sequences are separated by comma, no space)
Example for “Large-scale output version:” :
1. Prepare your xls, xlsx, txt or fasta files → → → 2. Upload the file through “Choose File” botton → → → 3. Select one or several models → → → 4. Click “Run” → → → 5. It will automatically download your results.
Notice: File preparation should follow the examples under this repository https://github.com/dzjxzyd/UniDL4BioPep_webserver/tree/main/Example%20uploading%20files
Detailed explaination of the activity abbreviation
Antihypertensive: Angiotensin-converting enzyme inhibitory activity (main target in for hypertension); DPPIV: dipeptidyl peptidase IV (DPPIV) inhibitory activity (main target for diabetes); AMP: antimicrobial activity; AMAP: antimalarial activity (main and alternative is corresponding to two datasets; QS: quorum-sensing activity; ACP: anticancer activity: Anti_MRSA: anti-methicillin-resistant S. aureus strains activity); TTCA: tumor T cell antigens; BBP: blood-brain barrier penetrating; APP: anti-parasitic activity;
The whole model architecture
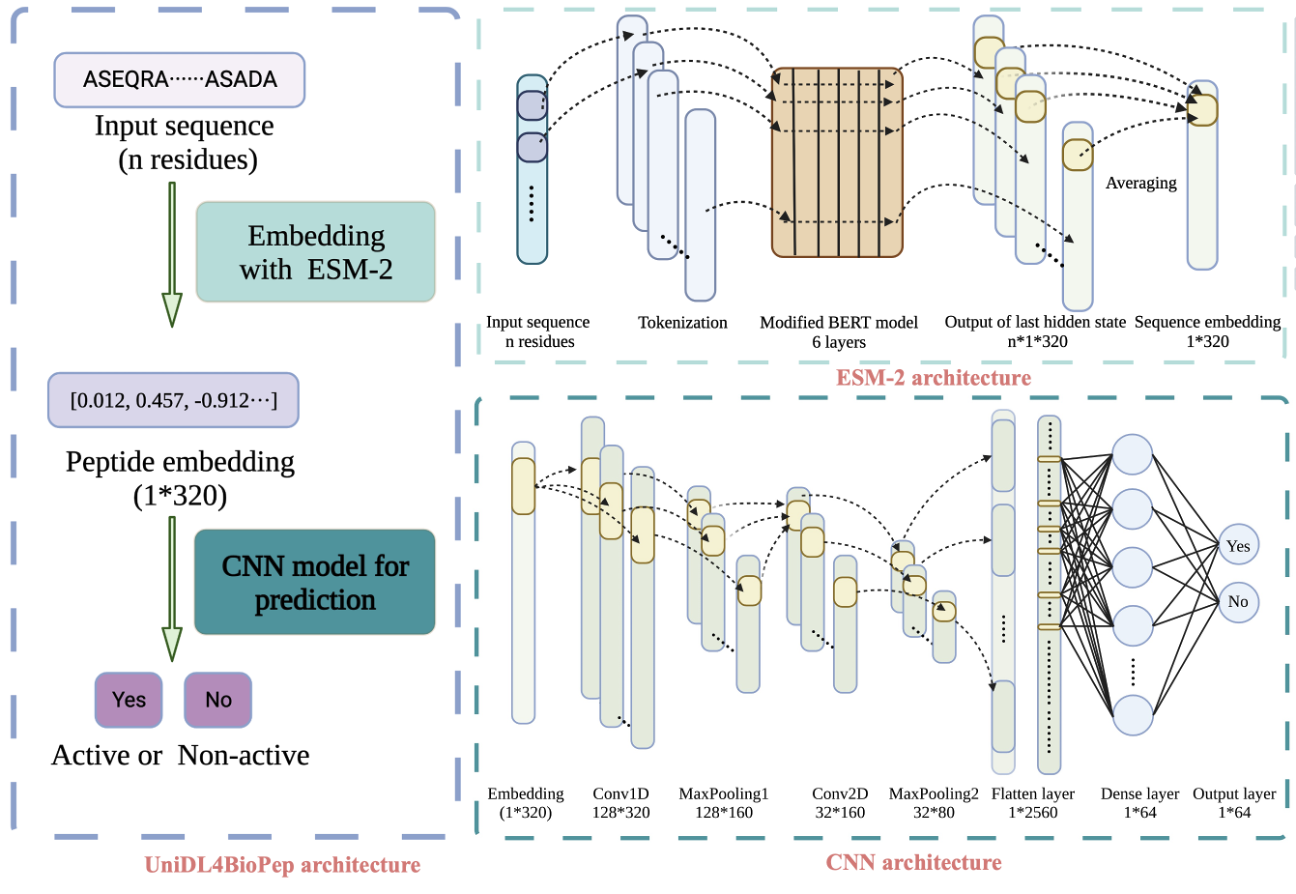
Dataset for the those models
Bioactivity | Training dataset | Test dataset |
---|---|---|
ACE inhibitory activity | 913 positives and 913 negatives | 386 positives and 386 negatives |
DPP IV inhibitory activity | 532 positives and 532 negatives | 133 positives and 133 negatives |
Bitter | 256 positives and 256 negatives | 64 positives and 64 negatives |
Umami | 112 positives and 241 negatives | 28 positives and 61 negatives |
Antimicrobial activity | 3876 positives and 9552 negatives | 2584 positives and 6369 negatives |
Antimalarial activity | Main dataset (111 positives and 1708 negatives); alternative dataset (111 positives and 542 negatives) | Main dataset (28 positives and 427 negatives); alternative dataset (28 positives and 135 negatives) |
Quorum sensing activity | 200 positives and 200 negatives | 20 positives and 20 negatives |
Anticancer activity | Main dataset (689 positives and 689 negatives); alternative dataset (776 positives and 776 negatives) | Main dataset (172 positives and 172 negatives); alternative dataset (194 positives and 194 negatives) |
Anti-MRSA strains activity | 118 positives and 678 negatives | 30 positives and 169 negatives |
Tumor T cell antigens | 470 positives and 318 negatives | 122 positives and 75 negatives |
Blood-Brain Barrier | 100 positives and 100 negatives | 19 positives and 19 negatives |
Anti-parasitic activity | 255 positives and 255 negatives | 46 positives and 46 negatives |
Neuropeptide | 1940 positives and 1940 negatives | 485 positives and 485 negatives |
Antibacterial activity | 6583 positives and 6583 negatives | 1695 positives and 1695 negatives | Antifungal activity | 778 positives and 778 negatives | 215 positives and 215 negatives |
Antiviral activity | 2321 positives and 2321 negatives | 623 positives and 623 negatives |
Toxicity | 1642 positives and 1642 negatives | 290 positives and 290 negatives |
Antioxidant activity | 582 positives and 541 negatives | 146 positives and 135 negatives |